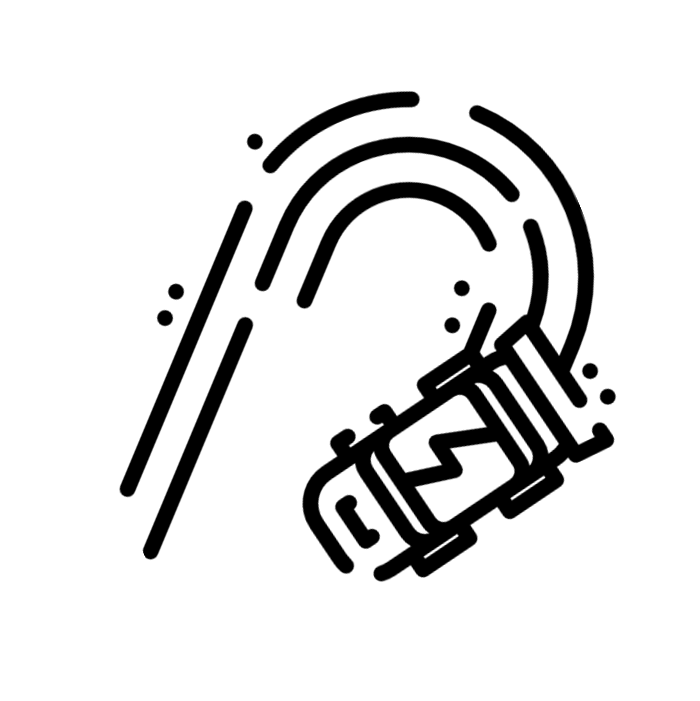
What is TKYO Drift?
AI models degrade over time due to data drift, concept drift, or external changes, leading to worse predictions without developers noticing. Many teams lack visibility into how AI models evolve in production. - oslabs-beta/tkyo-drift
Problem
Users struggle to monitor text-to-text AI model degradation over time due to data drift, concept drift, or external changes, leading to worse predictions without developers noticing and lack of visibility into model evolution.
Solution
An open-source monitoring tool that allows users to track drift in text-to-text AI models, detect performance degradation, and set alerts for anomalies. Example: Integrate with production models to visualize metrics and trigger notifications.
Customers
Data scientists, machine learning engineers, and AI development teams managing text-based models in production environments.
Unique Features
Specializes in text-to-text model drift detection, open-source flexibility, and real-time performance tracking with customizable thresholds.
User Comments
Simplifies model monitoring for NLP systems
Critical for maintaining reliable AI outputs
Open-source approach encourages collaboration
Reduces debugging time for unexpected model behavior
Lacks integration with some cloud platforms
Traction
Launched on ProductHunt with 500+ upvotes, 1.2k+ GitHub stars, and contributions from 50+ developers. Actively used by 200+ teams as per repository insights.
Market Size
The AI monitoring and observability market is projected to reach $4 billion by 2028 (MarketsandMarkets, 2023).