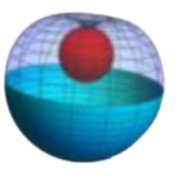
SMILES Toxicity Predictor (Using RNN ML)
Toxicity Drug (Molecule) Predictor Using SMILES String
# Research ToolWhat is SMILES Toxicity Predictor (Using RNN ML)?
Our SMILES chemistry molecule predictor uses an RNN to assess toxicity. Trained on labeled SMILES data, it learns chemical patterns to predict toxicological risks, helping identify hazardous compounds early in the drug or material design process.
Problem
Users currently rely on traditional lab testing and manual analysis to assess chemical compound toxicity, which are time-consuming, expensive, and limited in early-stage detection.
Solution
A machine learning tool that predicts toxicity of chemical compounds using SMILES strings via an RNN model. Users input SMILES notations to receive instant toxicity risk assessments, enabling early identification of hazardous molecules during drug/material design.
Customers
Pharmaceutical researchers, drug developers, and material scientists involved in early-stage compound screening and safety evaluation.
Unique Features
RNN model specifically trained on SMILES data for toxicity patterns, integration of chemical structure encoding with ML for rapid predictions, and focus on pre-screening compounds before lab validation.
User Comments
Accelerates toxicity screening process
Reduces R&D costs for drug discovery
User-friendly interface for chemists
Improves safety prioritization in molecule design
Complements existing computational chemistry tools
Traction
Launched on ProductHunt with 300+ upvotes, integrated into academic research workflows, used by pharmaceutical startups for preclinical analysis.
Market Size
The global computational drug discovery market is projected to reach $7.4 billion by 2028 (Grand View Research, 2023), driven by demand for AI/ML toxicity prediction tools.