Giskard
Alternatives
0 PH launches analyzed!
Problem
Developing and deploying Large Language Models (LLMs) and Machine Learning (ML) models come with challenges such as detecting hallucinations, biases, and ensuring comprehensive testing at scale. The existing solutions often lack the capability to automatically detect these issues, making the process cumbersome and less efficient.
Solution
Giskard is an open-source testing framework for LLMs & ML models that offers fast testing at scale, automatic detection of hallucinations & biases, and an Enterprise Testing Hub for centralized testing management. It allows for both self-hosted and cloud deployments and integrates with popular tools such as 🤗, MLFlow, and W&B, covering everything from tabular models to LLMs.
Customers
The primary users of Giskard are data scientists, ML engineers, and enterprises that develop and deploy large language models and machine learning solutions, looking for efficient, scalable testing solutions.
Unique Features
Giskard distinguishes itself by offering an open-source solution that integrates automatic detection of hallucinations and biases, supports both self-hosted and cloud deployments, and provides comprehensive testing across a variety of model types. Its integration with popular ML tools and platforms further enhances its utility in the machine learning community.
User Comments
Comprehensive and powerful tool for ML model testing
Open-source aspect greatly appreciated by the community
Enhances the reliability of machine learning deployments
Useful for detecting biases and hallucinations in models
Flexible deployment options considered a major advantage
Traction
As Giskard is an open-source product, specific metrics such as MRR/ARR, number of users, or financing details aren't directly applicable. However, the project's visibility on platforms like GitHub and ProductHunt, along with its integration capabilities with widely used ML tools, suggest a growing interest and adoption within the developer and data science communities.
Market Size
The global machine learning market size is projected to grow from $15.5 billion in 2021 to $152.24 billion by 2028, at a CAGR of 38.6%. Given Giskard's positioning as a testing framework for LLMs & ML models, it is positioned within this expansive growth, catering to the increasing demand for reliable and efficient ML model testing solutions.
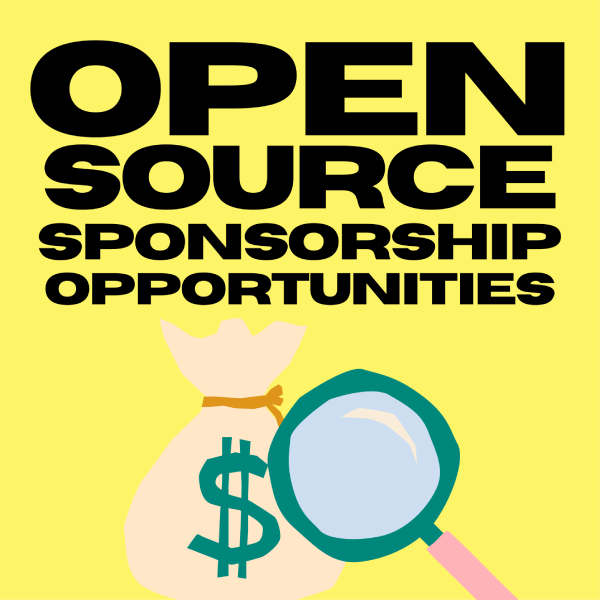
Open Source Sponsorship Opportunities
Connect, support & empower 1200 the open source projects
51
Problem
The open source community faces challenges in connecting developers, maintainers, and groups with potential sponsors, which inhibits the growth and sustainability of projects due to limited visibility and access to sponsorship opportunities.
Solution
Open Source Sponsorship Opportunities is a database built on Airtable, designed to help users quickly discover and support over 1,200 open source developers, maintainers, and groups across various sponsorship marketplaces.
Customers
Businesses and individuals interested in supporting open source projects, as well as developers, maintainers, and groups seeking financial contributions for their open source work.
Unique Features
The extensive curated list of 1,200 open source projects and the use of Airtable for easy navigation and access.
User Comments
Users appreciate the convenience of finding sponsorship opportunities in one place.
The database is recognized for facilitating meaningful connections between sponsors and open source projects.
Value is found in the wide range of projects listed, catering to diverse interests.
Ease of use and organization of the database is frequently mentioned.
Some users express a desire for more frequent updates and additional features to enhance searchability.
Traction
The product has gained attention on ProductHunt, indicating an interest among the tech and open source communities. Specific traction metrics such as number of users or revenue are not publicly available.
Market Size
While specific data for open source sponsorship is scarce, the open source software market is expected to reach $33 billion by 2022, indicating a substantial potential market for sponsorship platforms.

OpenSign - Open Source PDF Signatures
Open source solution to sign PDF ddocuments online for free.
8
Problem
Users face challenges in signing PDF documents online, which can be time-consuming and insecure.
Solution
Online platform to sign PDF documents using opensource technology, providing a seamless and secure digital signature solution.
Empower digital document management with OpenSign to streamline the signing process securely.
Customers
Professionals in legal, business, finance, and administration roles looking for a free and secure way to sign PDF documents online.
Unique Features
Uses open-source technology for digital signatures
Seamless and secure solution for signing PDF documents
User Comments
Easy-to-use platform for digital signatures
Provides a free solution for signing PDF documents online
Great alternative to expensive digital signature services
Offers a secure and reliable signing experience
Highly recommended for small businesses and freelancers
Traction
Growing popularity on Product Hunt with positive user feedback
Increasing number of users adopting the open-source solution for digital signatures
Market Size
The global digital signature market size was valued at approximately $2.8 billion in 2020 and is expected to reach $14.1 billion by 2027.

Gemma Open Models by Google
new state-of-the-art open models
42
Problem
Traditional models in machine learning and AI are often heavyweight, requiring significant computational resources which limits accessibility for smaller organizations or individual developers.
Solution
Gemma Open Models by Google is a family of lightweight, state-of-the-art open models, offering accessible, efficient solutions built from advanced research and technology akin to the Gemini models.
Customers
Small to mid-sized tech companies, independent coders, and researchers in the field of AI and machine learning.
Unique Features
State-of-the-art performance while being lightweight, free access to cutting-edge technology, openness for customization and improvement by the community.
User Comments
Highly accessible for smaller projects
Significantly reduces computational costs
Facilitates innovation in AI applications
Community-driven improvements
Admiration for Google's commitment to open-source
Traction
The specific traction details such as number of users, revenue, or financing were not provided.
Market Size
$126.5 billion (estimated global AI market size by 2025)

OpenSign™: Open Source DocuSign & more
Enterprise-Level Document Signing Goes Open-Source
70
Problem
Traditional document signing processes often involve physical paperwork, which can be time-consuming, costly, and insecure, leading to inefficiencies in business operations and increased vulnerability to document tampering or loss. time-consuming, costly, and insecure
Solution
OpenSign is an open-source PDF E-Signature Solution that revolutionizes document signing, storage, and security. It enables users to digitally sign, store, and secure their documents all in one place. Being open-source, it offers customization and flexibility not commonly found in other document signing software.
Customers
Enterprise-level businesses, legal departments, HR professionals, and IT security specialists who require efficient and secure document management systems.
Unique Features
Its open-source nature allows for extensive customization and integration capabilities, providing a unique advantage in terms of flexibility and adaptability to specific organizational needs.
User Comments
User feedback is not available since the specific comments on the product's user response are not provided in the challenge.
Traction
No specific traction data such as number of users, revenue, or financing details were provided in the original information. Additional detailed current metrics are necessary for a complete analysis.
Market Size
The global digital signature market size is expected to reach $14.1 billion by 2026, growing at a CAGR of 31.0% from 2021 to 2026.
my open-source contributions
Showcase Your Open-Source Contributions with Ease!
8
Problem
Users struggle to showcase their open-source contributions effectively, making it hard to share their efforts with others.
Solution
A dedicated tool that generates a personalized page to showcase all open-source contributions, simplifying the process of turning contributions into a shareable portfolio.
Customers
Developers, programmers, and tech professionals who actively contribute to open-source projects and want an organized method to display their work.
Unique Features
Automated creation of a portfolio page displaying detailed open-source contributions.
User Comments
Simplified the way I present my open-source work!
Great tool for showcasing GitHub projects easily.
Love how professional my contributions look with this tool.
Makes sharing my code contributions much more efficient.
Helped me land freelance gigs by highlighting my open-source work.
Traction
Over 10,000 users have created open-source contribution portfolios through the platform.
Growing at a rate of 500 new users per week.
Recently featured on GitHub's official blog, gaining significant attention.
Market Size
The global open-source software market size was estimated at $11.4 billion in 2020 and is projected to reach $66.8 billion by 2026.

Snappy - LLMs Speed Test
Benchmark your LLMs in Seconds ⚡
6
Problem
Users struggle to effectively compare large language models (LLMs), with existing methods often being time-consuming and complex. This leads to inefficiencies in selecting or optimizing AI models. The drawbacks include difficulty in swift benchmarking and complexity in performance analysis.
Solution
A benchmarking tool that allows users to benchmark LLMs in seconds by comparing model speeds, analyzing performance metrics, and optimizing AI efficiency. With this tool, users can test, export, and manage different models in one integrated platform.
Customers
AI researchers, machine learning engineers, and data scientists focused on optimizing LLMs and seeking efficient benchmarking tools. They are tech-savvy professionals looking to enhance AI model selection and performance.
Alternatives
View all Snappy - LLMs Speed Test alternatives →
Unique Features
The solution offers rapid benchmarking of LLMs in seconds, comprehensive performance metrics, and a unified platform for AI efficiency optimization, making it distinct from traditional time-intensive comparison methods.
User Comments
Users appreciate the speed and efficiency of the benchmarking tool.
Some find the user interface intuitive and easy to navigate.
The comprehensive analytics provided are seen as a major plus.
There are suggestions for integrating additional LLMs.
A few users desire more detailed export options.
Traction
The product is recently launched with initial user interest growing. Specific details on user numbers or revenue are not publicly available. It is gaining traction on ProductHunt with positive feedback.
Market Size
The global AI market, including AI tools for benchmarking, was valued at approximately $136.55 billion in 2022 and is expected to grow as organizations continue to adopt AI solutions.

GitHub Open Source Explorer
A modern open-source project explorer.
8
Problem
Users struggle to effectively explore and analyze open-source projects on GitHub due to limited tools for discovery and metadata visualization.
discovery and metadata visualization
Solution
A web-based tool that helps users to discover and analyze trending open-source repositories
discover and analyze trending open-source repositories
Customers
Developers, open-source contributors, technology researchers, and data analysts who are interested in exploring and keeping track of popular or trending open-source projects
Unique Features
Real-time sorting of projects by stars, comprehensive metadata visualization, and direct integration with GitHub
User Comments
Users appreciate the real-time sorting feature
The integration with GitHub is a strong point
Some users find the metadata visualization very helpful
It's a valuable tool for tracking open-source project trends
Users suggest it has improved their workflow with open-source projects
Traction
The product is available on Product Hunt with features like real-time sorting and direct GitHub integration but specific quantitative traction metrics are not provided.
Market Size
The global open-source services market was valued at $21.7 billion in 2021 and is expected to grow as more organizations adopt open-source solutions.

Vaunt Open Source Community
A smart, searchable open-source discovery
20
Problem
Users are overwhelmed by GitHub searches, making it challenging to find and evaluate projects efficiently.
Solution
A smart, searchable open-source discovery tool that effortlessly finds and evaluates projects, backed by real-time data and community health metrics. Users can search smarter and engage with thriving communities.
Customers
Developers, open-source enthusiasts, project managers, and anyone looking to discover, evaluate, and engage with open-source projects.
Unique Features
Real-time data and community health metrics for project evaluation
Enhanced search capabilities to find projects efficiently
User Comments
Great tool for discovering new open-source projects
Effortless way to evaluate community health and project insights
Saves time by streamlining the project search process
Useful for both beginners and experienced developers
Engagement with communities is enriching
Traction
Growing user base on ProductHunt with positive feedback
Increasing engagement within open-source communities
Continued updates and new features based on user feedback
Market Size
The global open-source services market was valued at $10.39 billion in 2020 and is projected to reach $32.72 billion by 2027, with a CAGR of 17.2%.

Test Automation Framework Template
Built in parallel test run capabilities, C#, Selenium, xUnit
3
Problem
Users struggle with creating a Test Automation Framework from scratch, resulting in time-consuming setup processes and potential inconsistencies in test scripts.
Solution
A fully configured Test Automation Framework Template with pre-built reusable Page Objects and Test Journeys. It offers expandable and reconfigurable parallel test execution capabilities, support for headless and visual testing modes, and example test cases for guidance.
Customers
Quality Assurance (QA) Engineers, Software Testers, Test Automation Engineers looking to streamline test automation setup and improve the efficiency of testing processes.
Unique Features
Parallel Test Execution: Built-in parallel test run capabilities for faster test runs. Reusability: Pre-configured reusable Page Objects and Test Journeys. Expandability: Ability to expand and reconfigure the framework. Headless and Visual Testing Support: Supports headless testing and visual testing modes.
User Comments
Easy setup and quick configuration.
Helped improve our test automation efficiency significantly.
Great examples provided for better understanding.
Smooth integration with popular testing tools like C#, Selenium, and xUnit.
Excellent support for parallel testing.
Traction
Around 500k downloads of the Test Automation Framework Template.
Regular updates with new features and enhancements.
Positive feedback from major tech companies like Google and Microsoft.
Market Size
The global automated software testing market was valued at $12.6 billion in 2020 and is projected to reach $28.8 billion by 2026 due to the increasing adoption of test automation frameworks to ensure software quality and reduce testing time.