ContextCheck
Alternatives
0 PH launches analyzed!

ContextCheck
Framework for testing and evaluating LLMs, RAG & chatbots.
11
Problem
Users face challenges in testing and evaluating Large Language Models (LLMs), Retrieval Augmented Generators (RAGs), and chatbots
Current methods lack tools to automate query generation, request completion, regression detection, penetration testing, and hallucination assessment
Solution
An open-source framework for testing LLMs, RAGs, and chatbots
Offers tools for automatic query generation, request completions, regression detection, penetration testing, and hallucination assessment to ensure system robustness and reliability
Customers
AI researchers
Data scientists
Developers working with LLMs, RAGs, and chatbots
Alternatives
Unique Features
Tools for automatic query generation and completion
Includes regression detection and penetration testing features
Focus on robustness and reliability assurance in LLMs, RAGs, and chatbots
User Comments
Useful for testing AI models
Great tool for ensuring the reliability of chatbots
Easy to use and implement
Helps in identifying system regressions
Improves the testing process significantly
Traction
Growing user base among AI researchers and data scientists
Positive feedback on new feature releases
Increasing adoption in the AI testing community
Market Size
The artificial intelligence testing market is projected to reach $28.8 billion by 2027
Increasing adoption of AI models drives the demand for testing frameworks

Test Automation Framework Template
Built in parallel test run capabilities, C#, Selenium, xUnit
3
Problem
Users struggle with creating a Test Automation Framework from scratch, resulting in time-consuming setup processes and potential inconsistencies in test scripts.
Solution
A fully configured Test Automation Framework Template with pre-built reusable Page Objects and Test Journeys. It offers expandable and reconfigurable parallel test execution capabilities, support for headless and visual testing modes, and example test cases for guidance.
Customers
Quality Assurance (QA) Engineers, Software Testers, Test Automation Engineers looking to streamline test automation setup and improve the efficiency of testing processes.
Unique Features
Parallel Test Execution: Built-in parallel test run capabilities for faster test runs. Reusability: Pre-configured reusable Page Objects and Test Journeys. Expandability: Ability to expand and reconfigure the framework. Headless and Visual Testing Support: Supports headless testing and visual testing modes.
User Comments
Easy setup and quick configuration.
Helped improve our test automation efficiency significantly.
Great examples provided for better understanding.
Smooth integration with popular testing tools like C#, Selenium, and xUnit.
Excellent support for parallel testing.
Traction
Around 500k downloads of the Test Automation Framework Template.
Regular updates with new features and enhancements.
Positive feedback from major tech companies like Google and Microsoft.
Market Size
The global automated software testing market was valued at $12.6 billion in 2020 and is projected to reach $28.8 billion by 2026 due to the increasing adoption of test automation frameworks to ensure software quality and reduce testing time.

Specialized Custom Chatbot AI
AI, chatbot, RAG, graph RAG, RAG models, customization
8
Problem
Users struggle to deploy chatbots that are specifically tailored to their unique data sources, which limits the effectiveness of their customer interactions.
Deploy chatbots that are specifically tailored to their unique data sources
Solution
On-premise RAG based chatbot
Customize RAG models, chain them together for even better results and response
With this product, users can create and deploy chatbots that effectively utilize their own dataset, resulting in more precise and customized user interactions.
Customers
IT managers, AI developers, and data scientists
who are looking for robust and customizable AI solutions for customer service.
These users typically are responsible for managing company-specific data and require tools that offer customization and localization.
Unique Features
Integration with Graph RAG to enhance response quality
Ability to customize and chain RAG models for improved chatbot performance
User Comments
Users appreciate the customization capability afforded by the product.
The integration with specific data sources is seen as invaluable.
Users find the chatbot's response more accurate and contextually relevant.
There is positive feedback regarding the on-premise deployment option for data security.
Users suggest slight improvements in user interface for greater ease of use.
Traction
Newly launched features include Graph RAG integration and customizable RAG models.
The number of early adopters is growing, indicating positive market reception.
Market Size
The global chatbot market was valued at approximately $2.6 billion in 2022 and is expected to expand at a CAGR of 25.7% from 2023 to 2030.

Snappy - LLMs Speed Test
Benchmark your LLMs in Seconds ⚡
6
Problem
Users struggle to effectively compare large language models (LLMs), with existing methods often being time-consuming and complex. This leads to inefficiencies in selecting or optimizing AI models. The drawbacks include difficulty in swift benchmarking and complexity in performance analysis.
Solution
A benchmarking tool that allows users to benchmark LLMs in seconds by comparing model speeds, analyzing performance metrics, and optimizing AI efficiency. With this tool, users can test, export, and manage different models in one integrated platform.
Customers
AI researchers, machine learning engineers, and data scientists focused on optimizing LLMs and seeking efficient benchmarking tools. They are tech-savvy professionals looking to enhance AI model selection and performance.
Alternatives
View all Snappy - LLMs Speed Test alternatives →
Unique Features
The solution offers rapid benchmarking of LLMs in seconds, comprehensive performance metrics, and a unified platform for AI efficiency optimization, making it distinct from traditional time-intensive comparison methods.
User Comments
Users appreciate the speed and efficiency of the benchmarking tool.
Some find the user interface intuitive and easy to navigate.
The comprehensive analytics provided are seen as a major plus.
There are suggestions for integrating additional LLMs.
A few users desire more detailed export options.
Traction
The product is recently launched with initial user interest growing. Specific details on user numbers or revenue are not publicly available. It is gaining traction on ProductHunt with positive feedback.
Market Size
The global AI market, including AI tools for benchmarking, was valued at approximately $136.55 billion in 2022 and is expected to grow as organizations continue to adopt AI solutions.
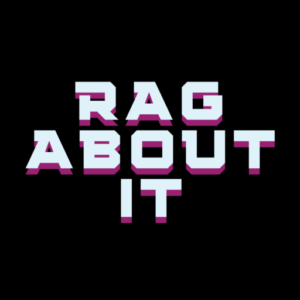
Rag About It
Dive deep into AI Retrieval Augmented Generation (RAG)
44
Problem
Users seeking to understand and apply AI Retrieval Augmented Generation (RAG) face a lack of centralized resources and difficulty in keeping up with the latest developments and technical knowledge in the field, leading to fragmented learning experiences and potential gaps in understanding.
Solution
Rag About It is a platform focused on providing comprehensive insights into AI Retrieval Augmented Generation (RAG), allowing users to explore recent advancements, technical knowledge, and applications of RAG systems through a dedicated resource.
Customers
Researchers, AI enthusiasts, practitioners in the field of artificial intelligence, and technology students.
Unique Features
Dedicated focus on RAG technology, centralization of technical knowledge and advancements, and support for a community interested in the specific niche of AI Retrieval Augmented Generation.
User Comments
Not available due to the nature of the question format.
Traction
Not available due to the nature of the question format.
Market Size
The global AI market size is projected to grow from $58.3 billion in 2021 to more than $309.6 billion by 2026.
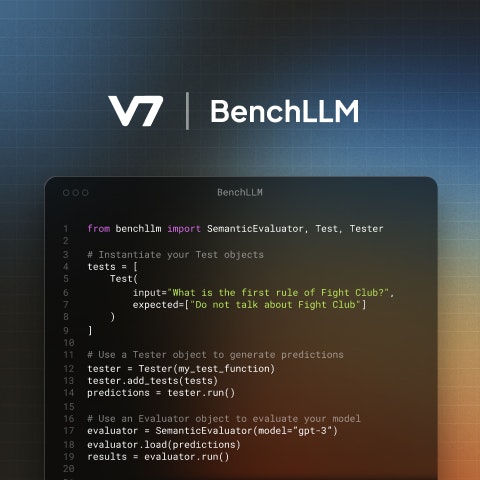
BenchLLM by V7
Test-driven development for LLMs
134
Problem
Developers and AI researchers traditionally spend significant time and resources manually testing large language models (LLMs) and chatbots to ensure they respond correctly to various prompts. This testing process is often labor-intensive, inefficient, and lacks scalability, making it difficult to test hundreds of prompts and responses on the fly.
Solution
BenchLLM is an open-source tool designed for test-driven development for LLMs, offering an efficient way to automate the testing process for LLMs, chatbots, and other AI-powered applications. Users can automate evaluations and benchmark models to build better and safer AI, simplifying the process of testing hundreds of prompts and responses on the fly.
Customers
Developers and AI researchers working on large language models and chatbots, looking for efficient ways to test and improve their AI-driven applications.
Alternatives
View all BenchLLM by V7 alternatives →
Unique Features
BenchLLM's key distinctive features include its ability to automate evaluations and rapidly benchmark models, which is critical for building better and safer AI applications. The tool's open-source nature and focus on test-driven development cater specifically to the needs of AI development workflows.
User Comments
Since specific user comments are not provided, an assessment of user opinions cannot be made without direct access to user feedback or reviews.
Traction
As specific traction data regarding BenchLLM, such as users, revenue, or funding, is not available through the provided links or without direct access to additional sources, precise details about its market acceptance and growth cannot be evaluated.
Market Size
The global AI market, encompassing tools such as BenchLLM, was valued at $93.5 billion in 2021, with expectations to grow significantly as AI development and deployment accelerate across various industries.
Problem
The current situation and problem faced by users is not clearly defined due to limited information provided. As such, this step lacks sufficient data to provide an elaborate analysis.
Solution
Testing tool or product. Lack of detailed features or functionalities due to minimal description.
Customers
The precise user persona for the product is undefined. More details on demographics and user behavior are needed for a comprehensive analysis.
Unique Features
Unique features or approaches of the solution are unclear due to the lack of detail in the description provided.
User Comments
The product lacks sufficient user reviews or comments, making it difficult to summarize user thoughts accurately.
Without further user interaction data or comments, this step remains incomplete.
Traction
Information regarding product traction such as user numbers, revenue, or recent updates is unavailable.
Market Size
Specific market size data unavailable; hence current industry values or comparable statistics are needed to supplement missing information.

Create my test
Convert your content into a test in seconds
53
Problem
Users struggle to create practice tests efficiently for various topics which could impact learning and test performance.
Solution
Create My Test is a tool that leverages artificial intelligence to convert content into various types of tests, including matching questions, fill in the blanks, multiple choice, and true/false. This facilitates efficient study and practice test creation.
Customers
Students, educators, and professionals looking for a method to create practice tests for studying or teaching purposes.
Unique Features
The ability to instantly convert content into a variety of test types using AI, specifically catering to different study needs and subjects.
User Comments
User comments are not provided in the given information.
Traction
Traction details such as version, user count, revenue, or financing are not provided in the given information.
Market Size
The global e-learning market was valued at $250 billion in 2020 and is expected to reach $1 trillion by 2027.
Problem
Users rely on basic retrieval methods for generating information, which limits the depth and specificity of understanding and application.
Solution
An open-source framework that advances Retrieval-Augmented Generation
Customers
Alternatives
View all PIKE-RAG alternatives →
Unique Features
It goes beyond basic retrieval to allow extraction, understanding, and application of domain-specific knowledge.
User Comments
Users appreciate the open-source nature of the framework.
The ability to handle domain-specific knowledge is seen as a major advantage.
Some find the setup and implementation process complex.
It is considered cutting-edge in the field of RAG.
Users are keen on continuous updates and improvements from Microsoft.
Traction
As of the current data, specific user numbers or revenue details are not publicly available.
Market Size
The AI and machine learning market was valued at $62 billion in 2021 and is expected to grow at a CAGR of 40.2% from 2022 to 2030.